ENHANCE
Project description
# theanonSR
Super Resolution using Deep Convolutional Neural Network(SRCNN) using theano
## Introduction
theanonSR upscales photo image to x2 size.
Original image
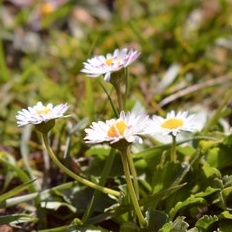
Upscaled image using python OpenCV library
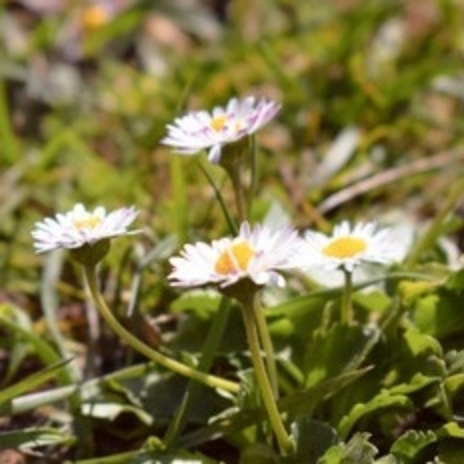
**Upscaled image using theanonSR**
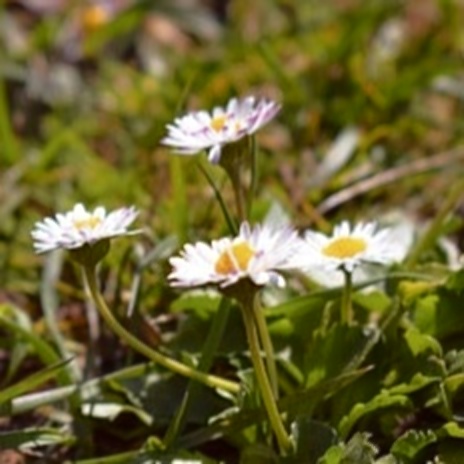
## Description
It is developed on python using theano library.
This project is to understand/study how deep convolutional neural network works
to learn super resolution of the image.
[TODO] Currently GPU support is not implemented yet.
## References
Originally, I was inspired this project from waifu2x project, which uses Torch7 to implement SRCNN.
- Chao Dong, Chen Change Loy, Kaiming He, Xiaoou Tang, "Image Super-Resolution Using Deep Convolutional Networks",
[http://arxiv.org/abs/1501.00092](http://arxiv.org/abs/1501.00092)
SRCNN, super resolution using deep convolutional neural network, is introduced in this paper.
- [waifu2x](https://github.com/nagadomi/waifu2x)
It is the popular project for image super resolution for Anime-Style art.
It also has a good performance.
- [theano](http://deeplearning.net/software/theano/index.html)
Machine learning library which can be written in python.
It also provides nice
[Deep Learning Tutorials](http://deeplearning.net/tutorial/) to study how to implement deep neural network.
## How to use
### Basic usage
Just specify image file path which you want to upscale.
Ex. Upscaling input.jpg
```
python code/srcnn.py input.jpg
```
### Specify output file name and path
Ex. Upscaling /path/to/input.jpg to /path/to/output.jpg
```
python code/srcnn.py /path/to/input.jpg /path/to/output.jpg
```
### Specify model to use:
You can specify directory name in the /model directory, as the model.
Ex. use model 32x3x3_32x3x3_32x3x3_1x3x3,
```
python code/srcnn.py -m 32x3x3_32x3x3_32x3x3_1x3x3 input.jpg
```
## Training
You can construct your own convolutional neural network, and train it easily!
### 1. Data preparation
Put training images[1] inside data/training_images directory.
(I used 2000 photo images during the training.)
[1]: Currently, image must be more than or equal to the size 232 x 232.
### 2. Construct your model (convolutional neural network)
Open code/tools/generate_model.py, and modify this code to construct your own model.
Then execute it.
```
python code/tools/generate_model.py
```
It will generate train.json file for your own model at model/your_model directory.
### 3. Training the model
Once prepared your own model to be trained, you can train your model by
```
python code/train_srcnn.py -m your_own_model
```
train_srcnn.py refers model/your_own_model/train.json to construct CNN (Convolutional Neural Network)
for training.
## Contribution is welcome
The performance of SR for this project is not matured.
You are welcome to improve & contribute this project.
If you could get any model which performs better performance, feel free to send me a pull request!
Super Resolution using Deep Convolutional Neural Network(SRCNN) using theano
## Introduction
theanonSR upscales photo image to x2 size.
Original image
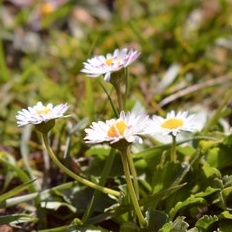
Upscaled image using python OpenCV library
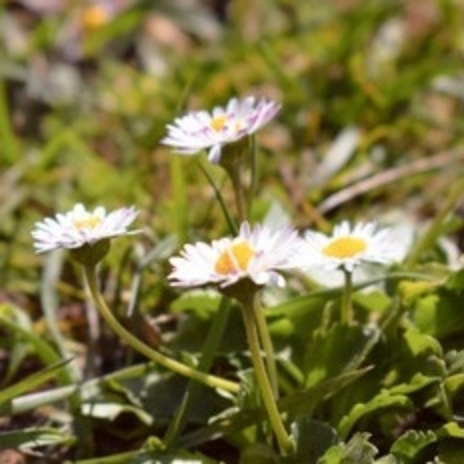
**Upscaled image using theanonSR**
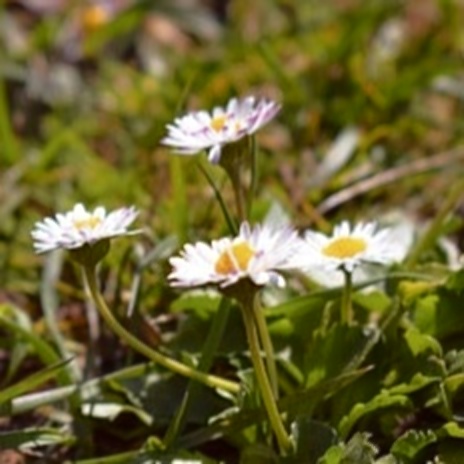
## Description
It is developed on python using theano library.
This project is to understand/study how deep convolutional neural network works
to learn super resolution of the image.
[TODO] Currently GPU support is not implemented yet.
## References
Originally, I was inspired this project from waifu2x project, which uses Torch7 to implement SRCNN.
- Chao Dong, Chen Change Loy, Kaiming He, Xiaoou Tang, "Image Super-Resolution Using Deep Convolutional Networks",
[http://arxiv.org/abs/1501.00092](http://arxiv.org/abs/1501.00092)
SRCNN, super resolution using deep convolutional neural network, is introduced in this paper.
- [waifu2x](https://github.com/nagadomi/waifu2x)
It is the popular project for image super resolution for Anime-Style art.
It also has a good performance.
- [theano](http://deeplearning.net/software/theano/index.html)
Machine learning library which can be written in python.
It also provides nice
[Deep Learning Tutorials](http://deeplearning.net/tutorial/) to study how to implement deep neural network.
## How to use
### Basic usage
Just specify image file path which you want to upscale.
Ex. Upscaling input.jpg
```
python code/srcnn.py input.jpg
```
### Specify output file name and path
Ex. Upscaling /path/to/input.jpg to /path/to/output.jpg
```
python code/srcnn.py /path/to/input.jpg /path/to/output.jpg
```
### Specify model to use:
You can specify directory name in the /model directory, as the model.
Ex. use model 32x3x3_32x3x3_32x3x3_1x3x3,
```
python code/srcnn.py -m 32x3x3_32x3x3_32x3x3_1x3x3 input.jpg
```
## Training
You can construct your own convolutional neural network, and train it easily!
### 1. Data preparation
Put training images[1] inside data/training_images directory.
(I used 2000 photo images during the training.)
[1]: Currently, image must be more than or equal to the size 232 x 232.
### 2. Construct your model (convolutional neural network)
Open code/tools/generate_model.py, and modify this code to construct your own model.
Then execute it.
```
python code/tools/generate_model.py
```
It will generate train.json file for your own model at model/your_model directory.
### 3. Training the model
Once prepared your own model to be trained, you can train your model by
```
python code/train_srcnn.py -m your_own_model
```
train_srcnn.py refers model/your_own_model/train.json to construct CNN (Convolutional Neural Network)
for training.
## Contribution is welcome
The performance of SR for this project is not matured.
You are welcome to improve & contribute this project.
If you could get any model which performs better performance, feel free to send me a pull request!
Project details
Release history Release notifications | RSS feed
Download files
Download the file for your platform. If you're not sure which to choose, learn more about installing packages.
Source Distribution
enhance-1.0.tar.gz
(9.0 kB
view hashes)