Introduction
In many machine learning applications, we often come across datasets where some types of data may be seen more than other types. Take identification of rare diseases for example, there are probably more normal samples than disease ones. In these cases, we need to make sure that the trained model is not biased towards the class that has more data. As an example, consider a dataset where there are 5 disease images and 20 normal images. If the model predicts all images to be normal, its accuracy is 80%, and F1-score of such a model is 0.88. Therefore, the model has high tendency to be biased toward the ‘normal’ class.
To solve this problem, a widely adopted technique is called resampling. It consists of removing samples from the majority class (under-sampling) and / or adding more examples from the minority class (over-sampling). Despite the advantage of balancing classes, these techniques also have their weaknesses (there is no free lunch). The simplest implementation of over-sampling is to duplicate random records from the minority class, which can cause overfitting. In under-sampling, the simplest technique involves removing random records from the majority class, which can cause loss of information.
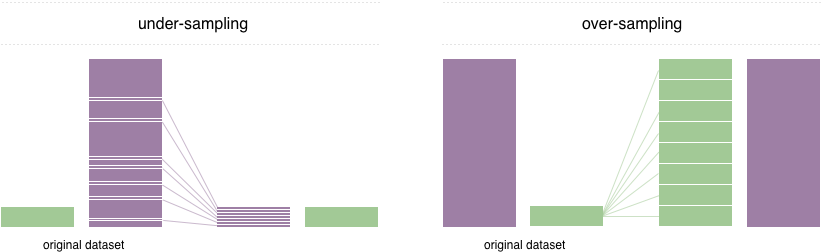
In this repo, we implement an easy-to-use PyTorch sampler ImbalancedDatasetSampler
that is able to
- rebalance the class distributions when sampling from the imbalanced dataset
- estimate the sampling weights automatically
- avoid creating a new balanced dataset
- mitigate overfitting when it is used in conjunction with data augmentation techniques
Usage
For a simple start install the package via one of following ways:
pip install torchsampler
Simply pass an ImbalancedDatasetSampler
for the parameter sampler
when creating a DataLoader
.
For example:
from torchsampler import ImbalancedDatasetSampler
train_loader = torch.utils.data.DataLoader(
train_dataset,
sampler=ImbalancedDatasetSampler(train_dataset),
batch_size=args.batch_size,
**kwargs
)
Then in each epoch, the loader will sample the entire dataset and weigh your samples inversely to your class appearing probability.
Example: Imbalanced MNIST Dataset
Distribution of classes in the imbalanced dataset:
With Imbalanced Dataset Sampler:
(left: test acc in each epoch; right: confusion matrix)
Without Imbalanced Dataset Sampler:
(left: test acc in each epoch; right: confusion matrix)
Note that there are significant improvements for minor classes such as 2
6
9
, while the accuracy of the other classes is preserved.
Contributing
We appreciate all contributions. If you are planning to contribute back bug-fixes, please do so without any further discussion. If you plan to contribute new features, utility functions or extensions, please first open an issue and discuss the feature with us.
Licensing