A DEep-learning and SIngle-cell based DEconvolution method for solid tumors
Project description
DeSide 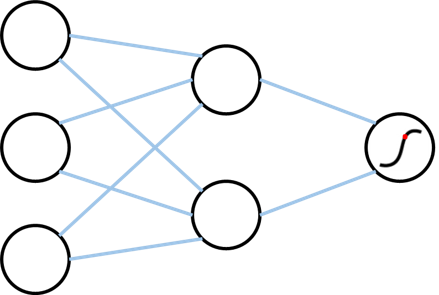
DeSide is a DEep-learning and SIngle-cell based DEconvolution method for solid tumors, which can be used to infer cellular proportions of different cell types from bulk RNA-seq data.
DeSide consists of the following four parts (see figure below):
- DNN Model
- Single Cell Dataset Integration
- Cell Proportion Generation
- Bulk Tumor Synthesis
In this repository, we provide the code for implementing these four parts and visualizing the results.
Requirements
DeSide requires Python 3.8 or higher. It has been tested on Linux and MacOS, but should work on Windows as well.
- tensorflow>=2.8.0
- scikit-learn==0.24.0
- anndata>=0.8.0
- scanpy==1.8.0
- pandas==1.2.5
- numpy<1.22
- matplotlib
- seaborn>=0.11.2
- bbknn==1.5.1
- SciencePlots
Installation
pip should work out of the box:
# create a virtual environment if necessary
conda create -n deside python=3.8
conda activate deside
pip install deside
Documentation
Documentation is available either in the source tree (doc/), or online. (will be available soon)
Usage Examples
Usage examples can be found: DeSide_mini_example
Three examples are provided:
- Using pre-trained model
- Training a model from scratch
- Generating a synthetic dataset
License
DeSide can be used under the terms of the MIT License.
Project details
Download files
Download the file for your platform. If you're not sure which to choose, learn more about installing packages.